Written by :
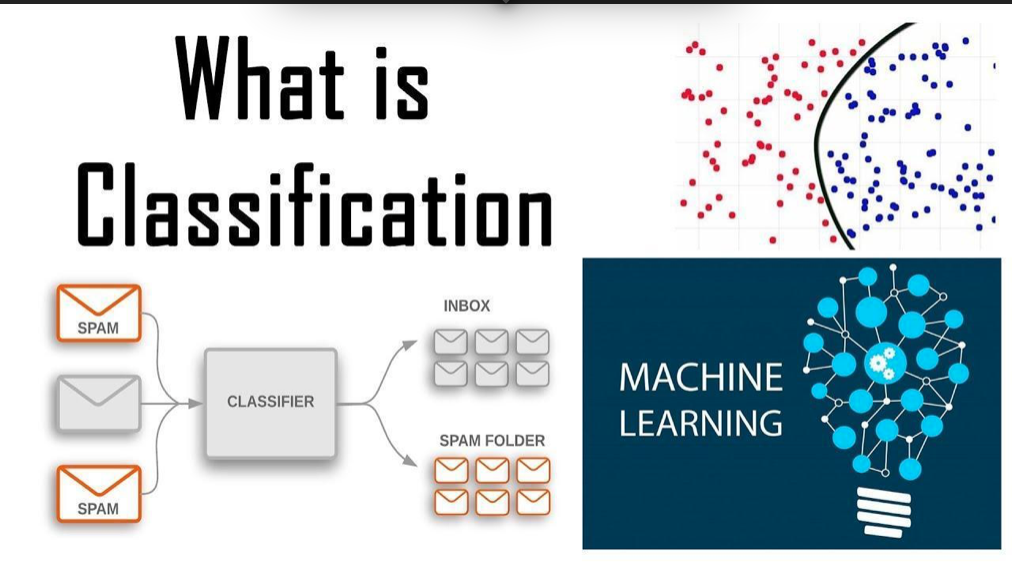
Classification in Machine Learning
What is classification?
Classification is defined as the process of recognition, understanding, and grouping of objects and ideas into preset categories a.k.a “sub-populations.” With the help of these pre-categorized training datasets, classification in machine learning programs leverage a wide range of algorithms to classify future datasets into respective and relevant categories.
Classification Predictive Modeling:
A classification problem in machine learning is one in which a class label is anticipated for a specific example of input data.
Problems with categorization include the following:
- Give an example and indicate whether it is spam or not.
- Identify a handwritten character as one of the recognized characters.
- Determine whether to label the current user behavior as churn
There are four different types of Classification Tasks in Machine Learning and they are following:
- Binary Classification
- Multi-Class Classification
- Multi-Label Classification
- Imbalanced Classification
Binary Classification:Those classification jobs with only two class labels are referred to as binary classification.
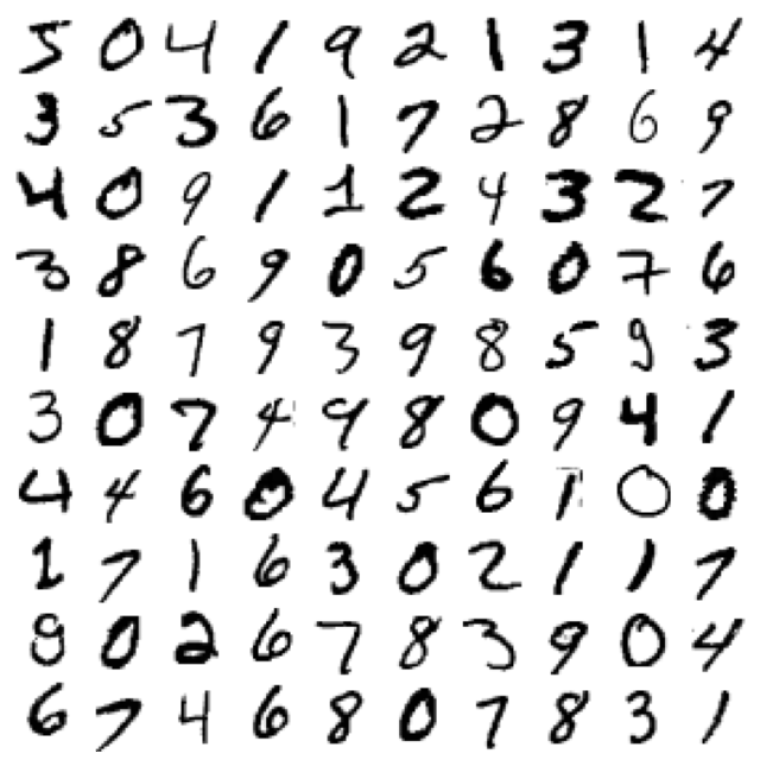
Examples:
- Prediction of conversion
- Churn forecast
- Detection of spam email
The following are well-known binary classification algorithms:
- Logistic Regression
- Support Vector Machines
- Simple Bayes
- Decision Trees
Multi-Class Classification:Multi-class labels are used in classification tasks referred to as multi-class classification.
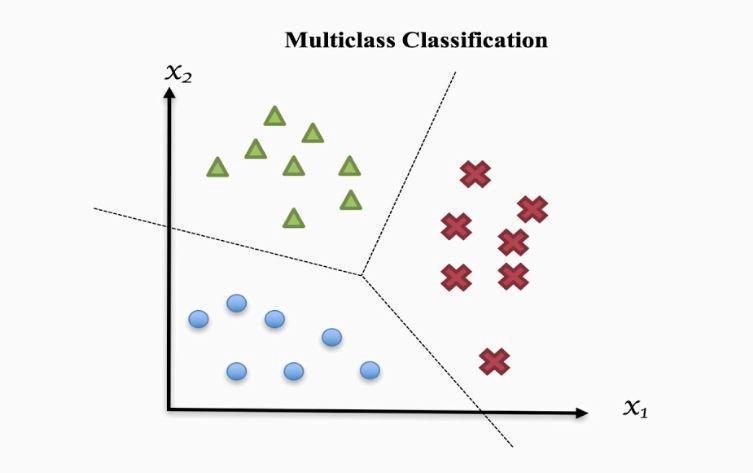
Examples:
- Categorization of faces.
- Classifying plant species.
- Character recognition using optical.
Multiclass classification tasks are frequently modeled using a model that forecasts a Multinoulli probability distribution for each example. For multi-class classification, many binary classification techniques are applicable.
The following well-known algorithms can be used for multi-class classification:
- Progressive Boosting
- Choice trees
- Nearest K Neighbors
- Rough Forest
- Simple Bayes
Multi-Label Classification:
Multi-label classification problems are those that feature two or more class labels and allow for the prediction
This greatly contrasts with multi-class classification and binary classification, which anticipate a single class label for each occurrence.
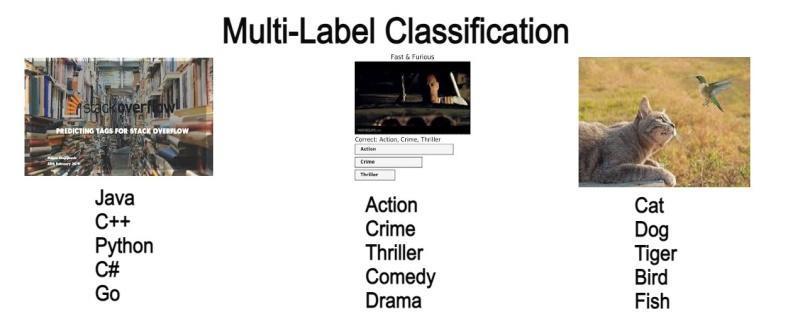
conventional classification algorithms:
- Multi-label Gradient Boosting
- Multi-label Random Forests
- Multi-label Decision Trees
Imbalanced Classification:
The term "imbalanced classification" describes classification jobs where the distribution of examples within each class is not equal.
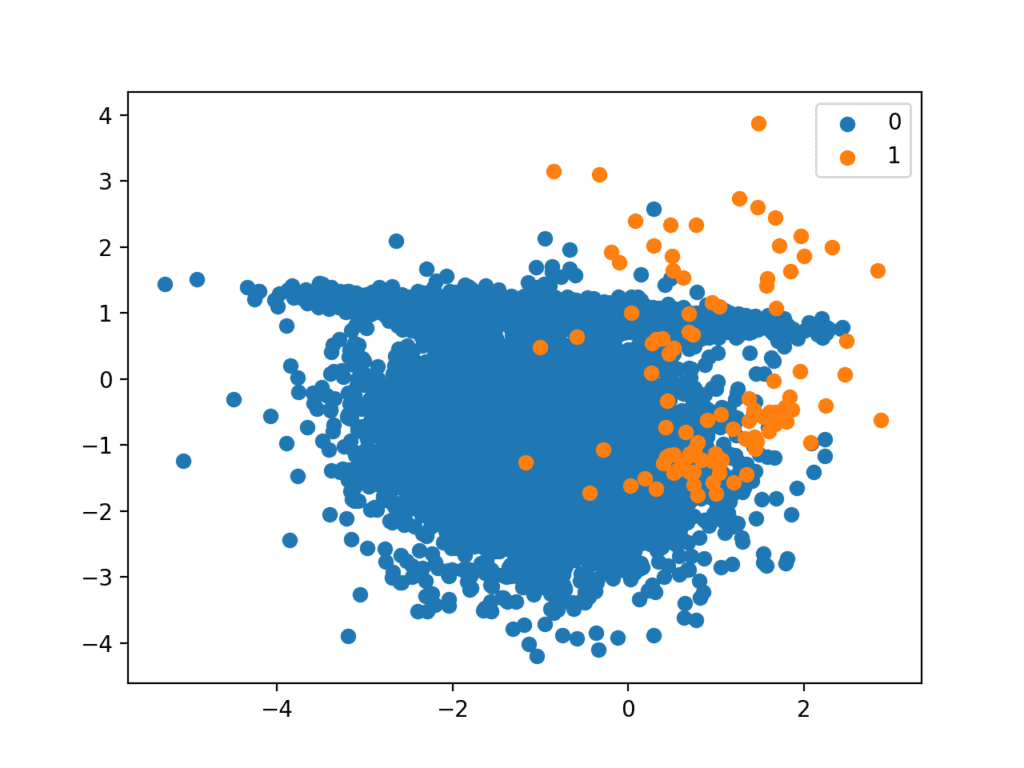
Examples:
- Clinical diagnostic procedures
- Detection of outliers
- Fraud investigation
Use Cases Of Classification Algorithms:
Different situations call for the usage of classification methods. Here are a few frequent applications for classification algorithms:
- Drugs Classification
- Email Spam Detection
- Identifications of Cancer tumor cells
- Biometric Identification, etc
- Speech Recognition